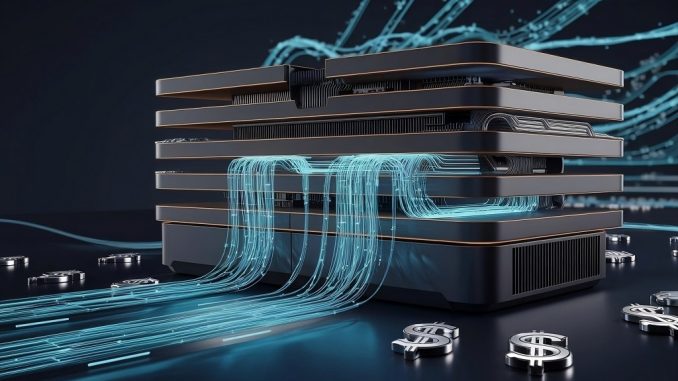
Join our daily and weekly newsletters for the latest updates and exclusive content on industry-leading AI coverage. Learn More
Graphics chips, or GPUs, are the engines of the AI revolution, powering the large language models (LLMs) that underpin chatbots and other AI applications. With price tags for these chips likely to fluctuate significantly in the years ahead, many businesses will need to learn how to manage variable costs for a critical product for the first time.
This is a discipline that some industries are already familiar with. Companies in energy-intensive sectors such as mining are used to managing fluctuating costs for energy, balancing different energy sources to achieve the right combination of availability and price. Logistics companies do this for shipping costs, which are vacillating wildly right now thanks to disruption in the Suez and Panama canals.
Volitivity ahead: The compute cost conundrum
Compute cost volatility is different because it will affect industries that have no experience with this type of cost management. Financial services and pharmaceutical companies, for example, don’t usually engage in energy or shipping trading, but they are among the companies that stand to benefit greatly from AI. They will need to learn fast.
Nvidia is the main provider of GPUs, which explains why its valuation soared this year. GPUs are prized because they can process many calculations in parallel, making them ideal for training and deploying LLMs. Nvidia’s chips have been so sought after that one company has had them delivered by armored car.
The costs associated with GPUs are likely to continue to fluctuate significantly and will be hard to anticipate, buffeted by the fundamentals of supply and demand.
Drivers of GPU cost volitivity
Demand is almost certain to increase as companies continue to build AI at a rapid pace. Investment firm Mizuho has said the total market for GPUs could grow tenfold over the next five years to more than $400 billion, as businesses rush to deploy new AI applications.
Supply depends on several factors that are hard to predict. They include manufacturing capacity, which is costly to scale, as well as geopolitical considerations — many GPUs are manufactured in Taiwan, whose continued independence is threatened by China.
Supplies have already been scarce, with some companies reportedly waiting six months to get their hands on Nvidia’s powerful H100 chips. As businesses become more dependent on GPUs to power AI applications, these dynamics mean that they will need to get to grips with managing variable costs.
Strategies for GPU cost management
To lock in costs, more companies may choose to manage their own GPU servers rather than renting them from cloud providers. This creates additional overhead but provides greater control and can lead to lower costs in the longer term. Companies may also buy up GPUs defensively: Even if they don’t know how they’ll use them yet, these defensive contracts can ensure they’ll have access to GPUs for future needs — and that their competitors won’t.
Not all GPUs are alike, so companies should optimize costs by securing the right type of GPUs for their intended purpose. The most powerful GPUs are most relevant for the handful of organizations that train giant foundational models, like OpenAI’s GPT and Meta’s LLama. Most companies will be doing less demanding, higher volume inference work, which involves running data against an existing model, for which a greater number of lower performance GPUs would be the right strategy.
Geographic location is another lever organizations can use to manage costs. GPUs are power hungry, and a large part of their unit economics is the cost of the electricity used to power them. Locating GPU servers in a region with access to cheap, abundant power, such as Norway, can significantly reduce costs compared to a region like the eastern U.S., where electricity costs are typically higher.
CIOs should also look closely at the trade-offs between the cost and quality of AI applications to strike the most effective balance. They may be able to use less computing power to run models for applications that demand less accuracy, for example, or that aren’t as strategic to their business.
Switching between different cloud service providers and different AI models provides a further way for organizations to optimize costs, much as logistics companies use different transport modes and shipping routes to manage costs today. They can also adopt technologies that optimize the cost of operating LLM models for different use cases, making GPU usage more efficient.
The challenge of demand forecasting
The whole field of AI computing continues to advance quickly, making it hard for organizations to forecast their own GPU demand accurately. Vendors are building newer LLMs that have more efficient architectures, like Mistral’s “Mixture-of-Experts” design, which requires only parts of a model to be used for different tasks. Chip makers including Nvidia and TitanML, meanwhile, are working on techniques to make inference more efficient.
At the same time, new applications and use cases are emerging that add to the challenge of predicting demand accurately. Even relatively simple use cases today, like RAG chatbots, may see changes in how they’re built, pushing GPU demand up or down. Predicting GPU demand is uncharted territory for most companies and will be hard to get it right.
Start planning for volatile GPU costs now
The surge in AI development shows no signs of abating. Global revenue associated with AI software, hardware, service and sales will grow 19% per year through 2026 to hit $900 billion, according to Bank of America Global Research and IDC. This is great news for chip makers like Nvidia, but for many businesses it will require learning a whole new discipline of cost management. They should start planning now.
Florian Douetteau is the CEO and co-founder of Dataiku.
DataDecisionMakers
Welcome to the VentureBeat community!
DataDecisionMakers is where experts, including the technical people doing data work, can share data-related insights and innovation.
If you want to read about cutting-edge ideas and up-to-date information, best practices, and the future of data and data tech, join us at DataDecisionMakers.
You might even consider contributing an article of your own!
Read More From DataDecisionMakers
Be the first to comment