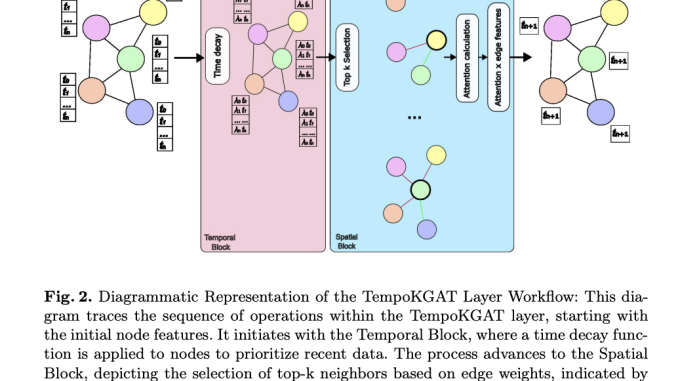
GNNs have excelled in analyzing structured data but face challenges with dynamic, temporal graphs. Traditional forecasting, often used in fields like economics and biology, relied on statistical models for time-series data. Deep learning, particularly GNNs, shifted focus to non-Euclidean data like social and biological networks. However, applying GNNs to dynamic graphs, where relationships constantly evolve, still needs to be improved. Although Graph Attention Networks (GATs) partially address these challenges, further advancements are needed, particularly in utilizing edge attributes.
Researchers from Sorbonne University and TotalEnergies have developed a graph attention network called TempoKGAT, which integrates time-decaying weights and a selective neighbor aggregation mechanism to uncover latent patterns in spatio-temporal graph data. This approach involves top-k neighbor selection based on edge weights, enhancing the representation of evolving graph features. TempoKGAT was tested on datasets from traffic, energy, and health sectors, consistently outperforming existing state-of-the-art methods across multiple metrics. These findings demonstrate TempoKGAT’s ability to improve prediction accuracy and provide deeper insights into temporal graph analysis.
Forecasting has evolved from traditional statistical methods to advanced machine learning, increasingly utilizing graph-based approaches to capture spatial dependencies. This progression has led from CNNs to GCNs and Graph Attention Networks (GATs). While models like Diffusion Convolutional Recurrent Neural Networks (DCRNN) and Temporal Graph Convolutional Networks (TGCN) incorporate temporal dynamics, they often overlook the benefits of weighted edges. Existing advancements in edge modeling, particularly for static and multi-relational graphs, have yet to be fully adapted to temporal contexts. TempoKGAT aims to address this gap by enhancing edge weight utilization in temporal graph forecasting, thereby improving prediction accuracy and analysis of complex temporal data.
The TempoKGAT model enhances temporal graph analysis by refining node features through time-decaying weights and selective neighbor aggregation. Starting with node features, a temporal decay is applied to prioritize recent data, ensuring dynamic graphs are accurately represented. The model then selects the top-k most significant neighbors based on edge weights, focusing on the most relevant interactions. An attention mechanism computes attention coefficients, normalized and used to aggregate neighbor features, weighted by attention scores and edge strengths. This approach dynamically integrates temporal and spatial insights, improving prediction accuracy and capturing evolving graph patterns.
TempoKGAT demonstrates exceptional performance across various datasets by effectively integrating temporal and spatial dynamics in graph data. The model significantly improved over the original GAT, with notable gains in metrics like MAE, MSE, and RMSE, particularly in datasets like PedalMe, ChickenPox, and England Covid. The adaptability of TempoKGAT is highlighted by its optimal neighborhood size parameter (k), which enhances prediction accuracy. Consistent success, especially at k = 1, underscores the model’s ability to capture critical features from immediate neighbors, making it a robust and versatile tool for graph-based predictive analytics across different network complexities.
In conclusion, TempoKGAT is a graph attention network designed for temporal graph analysis, which excels by integrating time-decaying weights and selective neighbor aggregation. The model outperforms traditional methods in predicting outcomes across datasets like PedalMe, ChickenPox, and England Covid, showing significant improvements in RMSE, MAE, and MSE metrics. However, the computational complexity increases with larger neighborhood sizes. Future research will optimize computational efficiency, explore multi-head attention, and scale the model for larger graphs, paving the way for broader applications in graph-based predictive analytics.
Check out the Paper. All credit for this research goes to the researchers of this project. Also, don’t forget to follow us on Twitter and LinkedIn. Join our Telegram Channel.
If you like our work, you will love our newsletter..
Don’t Forget to join our 50k+ ML SubReddit
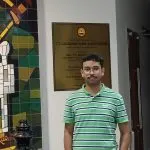
Sana Hassan, a consulting intern at Marktechpost and dual-degree student at IIT Madras, is passionate about applying technology and AI to address real-world challenges. With a keen interest in solving practical problems, he brings a fresh perspective to the intersection of AI and real-life solutions.
Be the first to comment