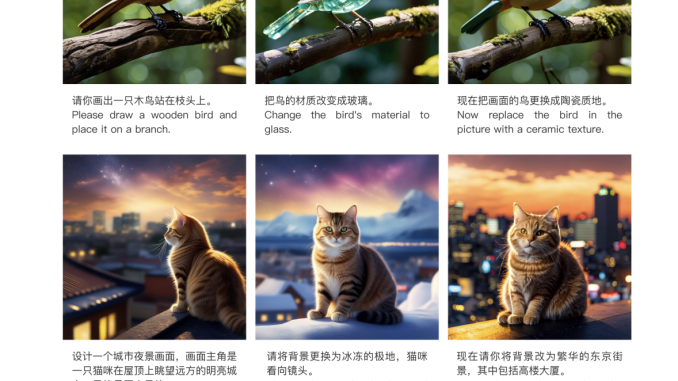
In recent research, a text-to-image diffusion transformer called Hunyuan-DiT has been developed with the goal of comprehending both English and Chinese text prompts in a subtle way. Several essential elements and procedures have been involved in the creation of Hunyuan-DiT in order to guarantee excellent picture production and fine-grained language comprehension.
The primary components of Hunyuan-DiT are as follows.
Transformer Structure: Hunyuan-DiTâs transformer architecture has been designed to maximize the modelâs ability to produce visuals from textual descriptions. This includes improving the modelâs ability to process intricate linguistic inputs and making sure it can record precise data.
Bilingual and Multilingual Encoding: Hunyuan-DiTâs ability to correctly read prompts is largely dependent on the text encoder. The model utilizes the strengths of both encoders, a bilingual CLIP that can handle both English and Chinese, and a multilingual T5 encoder in order to improve understanding and context handling.
Enhanced Positional Encoding: Hunyuan-DiTâs positional encoding algorithms have been adjusted to handle the sequential nature of text and the spatial characteristics of images more efficiently. This helps the model in correctly mapping tokens to appropriate image attributes and maintaining the token sequence.
The team has developed an extensive data pipeline that consists of the following components in order to enhance and support Hunyuan-DiTâs capabilities.
Data Curation and Collection: Assembling a sizable and varied dataset of text-image pairings.
Data augmentation and filtering: Adding more examples to the dataset and removing unnecessary or low-quality data.
Iterative Model Optimisation: Continuously updating and enhancing the modelâs performance based on fresh data and user feedback by employing the âdata convoyâ technique.
In order to improve the language understanding precision of the model, the team has specially trained an MLLM to improve the captions corresponding to the photos. By utilizing contextual knowledge, this model produces captions that are accurate and detailed, enhancing the quality of the images that are produced.
Hunyuan-DiT facilitates multi-turn dialogues that enable interactive image generation. This implies that over multiple iterations of engagement, people can offer input and improve the generated images, producing more accurate and pleasing outcomes.
To evaluate Hunyuan-DiT, the team has created a strict evaluation methodology with the participation of over 50 qualified evaluators. This technique measures the subject clarity, visual quality, lack of AI artifacts, text-image consistency, and other elements of the created images. Compared to other open-source models, the evaluations showed that Hunyuan-DiT delivers state-of-the-art performance in Chinese-to-image creation. It is excellent at creating crisp, semantically correct visuals in response to Chinese cues.Â
In conclusion, Hunyuan-DiT is a major breakthrough in text-to-image generation, especially for Chinese prompts. It provides outstanding performance in producing detailed and contextually accurate images by carefully constructing its transformer architecture, text encoders, and positional encoding, as well as by establishing a reliable data pipeline. Its capacity for interactive, multi-turn dialogues increases its usefulness even further, making it an effective tool for a range of uses.
Check out the Paper and GitHub. All credit for this research goes to the researchers of this project. Also, donât forget to follow us on Twitter. Join our Telegram Channel, Discord Channel, and LinkedIn Group.
If you like our work, you will love our newsletter..
Donât Forget to join our 42k+ ML SubReddit
Tanya Malhotra is a final year undergrad from the University of Petroleum & Energy Studies, Dehradun, pursuing BTech in Computer Science Engineering with a specialization in Artificial Intelligence and Machine Learning.She is a Data Science enthusiast with good analytical and critical thinking, along with an ardent interest in acquiring new skills, leading groups, and managing work in an organized manner.
Be the first to comment