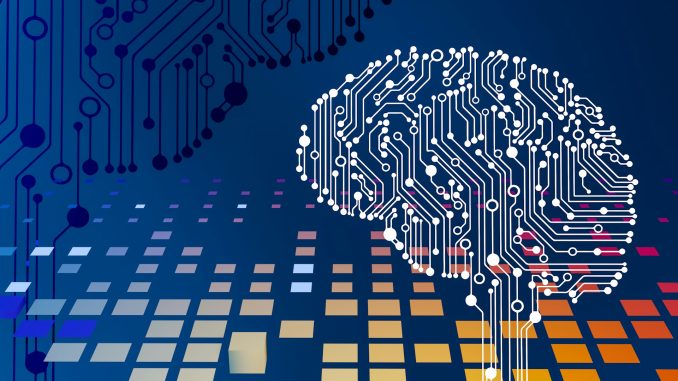
AI News caught up with president of Ikigai Labs, Kamal Ahluwalia, to discuss all things gen AI, including top tips on how to adopt and utilise the tech, and the importance of embedding ethics into AI design.
Could you tell us a little bit about Ikigai Labs and how it can help companies?
Ikigai is helping organisations transform sparse, siloed enterprise data into predictive and actionable insights with a generative AI platform specifically designed for structured, tabular data.
A significant portion of enterprise data is structured, tabular data, residing in systems like SAP and Salesforce. This data drives the planning and forecasting for an entire business. While there is a lot of excitement around Large Language Models (LLMs), which are great for unstructured data like text, Ikigai’s patented Large Graphical Models (LGMs), developed out of MIT, are focused on solving problems using structured data.
Ikigai’s solution focuses particularly on time-series datasets, as enterprises run on four key time series: sales, products, employees, and capital/cash. Understanding how these time series come together in critical moments, such as launching a new product or entering a new geography, is crucial for making better decisions that drive optimal outcomes.
How would you describe the current generative AI landscape, and how do you envision it developing in the future?
The technologies that have captured the imagination, such as LLMs from OpenAI, Anthropic, and others, come from a consumer background. They were trained on internet-scale data, and the training datasets are only getting larger, which requires significant computing power and storage. It took $100m to train GPT4, and GP5 is expected to cost $2.5bn.
This reality works in a consumer setting, where costs can be shared across a very large user set, and some mistakes are just part of the training process. But in the enterprise, mistakes cannot be tolerated, hallucinations are not an option, and accuracy is paramount. Additionally, the cost of training a model on internet-scale data is just not affordable, and companies that leverage a foundational model risk exposure of their IP and other sensitive data.
While some companies have gone the route of building their own tech stack so LLMs can be used in a safe environment, most organisations lack the talent and resources to build it themselves.
In spite of the challenges, enterprises want the kind of experience that LLMs provide. But the results need to be accurate – even when the data is sparse – and there must be a way to keep confidential data out of a foundational model. It’s also critical to find ways to lower the total cost of ownership, including the cost to train and upgrade the models, reliance on GPUs, and other issues related to governance and data retention. All of this leads to a very different set of solutions than what we currently have.
How can companies create a strategy to maximise the benefits of generative AI?
While much has been written about Large Language Models (LLMs) and their potential applications, many customers are asking “how do I build differentiation?”
With LLMs, nearly everyone will have access to the same capabilities, such as chatbot experiences or generating marketing emails and content – if everyone has the same use cases, it’s not a differentiator.
The key is to shift the focus from generic use cases to finding areas of optimisation and understanding specific to your business and circumstances. For example, if you’re in manufacturing and need to move operations out of China, how do you plan for uncertainty in logistics, labour, and other factors? Or, if you want to build more eco-friendly products, materials, vendors, and cost structures will change. How do you model this?
These use cases are some of the ways companies are attempting to use AI to run their business and plan in an uncertain world. Finding specificity and tailoring the technology to your unique needs is probably the best way to use AI to find true competitive advantage.
What are the main challenges companies face when deploying generative AI and how can these be overcome?
Listening to customers, we’ve learned that while many have experimented with generative AI, only a fraction have pushed things through to production due to prohibitive costs and security concerns. But what if your models could be trained just on your own data, running on CPUs rather than requiring GPUs, with accurate results and transparency around how you’re getting those results? What if all the regulatory and compliance issues were addressed, leaving no questions about where the data came from or how much data is being retrained? This is what Ikigai is bringing to the table with Large Graphical Models.
One challenge we’ve helped businesses address is the data problem. Nearly 100% of organisations are working with limited or imperfect data, and in many cases, this is a barrier to doing anything with AI. Companies often talk about data clean-up, but in reality, waiting for perfect data can hinder progress. AI solutions that can work with limited, sparse data are essential, as they allow companies to learn from what they have and account for change management.
The other challenge is how internal teams can partner with the technology for better outcomes. Especially in regulated industries, human oversight, validation, and reinforcement learning are necessary. Adding an expert in the loop ensures that AI is not making decisions in a vacuum, so finding solutions that incorporate human expertise is key.
To what extent do you think adopting generative AI successfully requires a shift in company culture and mindset?
Successfully adopting generative AI requires a significant shift in company culture and mindset, with strong commitment from executive and continuous education. I saw this firsthand at Eightfold when we were bringing our AI platform to companies in over 140 countries. I always recommend that teams first educate executives on what’s possible, how to do it, and how to get there. They need to have the commitment to see it through, which involves some experimentation and some committed course of action. They must also understand the expectations placed on colleagues, so they can be prepared for AI becoming a part of daily life.
Top-down commitment, and communication from executives goes a long way, as there’s a lot of fear-mongering suggesting that AI will take jobs, and executives need to set the tone that, while AI won’t eliminate jobs outright, everyone’s job is going to change in the next couple of years, not just for people at the bottom or middle levels, but for everyone. Ongoing education throughout the deployment is key for teams learning how to get value from the tools, and adapt the way they work to incorporate the new skillsets.
It’s also important to adopt technologies that play to the reality of the enterprise. For example, you have to let go of the idea that you need to get all your data in order to take action. In time-series forecasting, by the time you’ve taken four quarters to clean up data, there’s more data available, and it’s probably a mess. If you keep waiting for perfect data, you won’t be able to use your data at all. So AI solutions that can work with limited, sparse data are crucial, as you have to be able to learn from what you have.
Another important aspect is adding an expert in the loop. It would be a mistake to assume AI is magic. There are a lot of decisions, especially in regulated industries, where you can’t have AI just make the decision. You need oversight, validation, and reinforcement learning – this is exactly how consumer solutions became so good.
Are there any case studies you could share with us regarding companies successfully utilising generative AI?
One interesting example is a Marketplace customer that is using us to rationalise their product catalogue. They’re looking to understand the optimal number of SKUs to carry, so they can reduce their inventory carrying costs while still meeting customer needs. Another partner does workforce planning, forecasting, and scheduling, using us for labour balancing in hospitals, retail, and hospitality companies. In their case, all their data is sitting in different systems, and they must bring it into one view so they can balance employee wellness with operational excellence. But because we can support a wide variety of use cases, we work with clients doing everything from forecasting product usage as part of a move to a consumption-based model, to fraud detection.
You recently launched an AI Ethics Council. What kind of people are on this council and what is its purpose?
Our AI Ethics Council is all about making sure that the AI technology we’re building is grounded in ethics and responsible design. It’s a core part of who we are as a company, and I’m humbled and honoured to be a part of it alongside such an impressive group of individuals. Our council includes luminaries like Dr. Munther Dahleh, the Founding Director of the Institute for Data Systems and Society (IDSS) and a Professor at MIT; Aram A. Gavoor, Associate Dean at George Washington University and a recognised scholar in administrative law and national security; Dr. Michael Kearns, the National Center Chair for Computer and Information Science at the University of Pennsylvania; and Dr. Michael I. Jordan, a Distinguished Professor at UC Berkeley in the Departments of Electrical Engineering and Computer Science, and Statistics. I am also honoured to serve on this council alongside these esteemed individuals.
The purpose of our AI Ethics Council is to tackle pressing ethical and security issues impacting AI development and usage. As AI rapidly becomes central to consumers and businesses across nearly every industry, we believe it is crucial to prioritise responsible development and cannot ignore the need for ethical considerations. The council will convene quarterly to discuss important topics such as AI governance, data minimisation, confidentiality, lawfulness, accuracy and more. Following each meeting, the council will publish recommendations for actions and next steps that organisations should consider moving forward. As part of Ikigai Labs’ commitment to ethical AI deployment and innovation, we will implement the action items recommended by the council.
Ikigai Labs raised $25m funding in August last year. How will this help develop the company, its offerings and, ultimately, your customers?
We have a strong foundation of research and innovation coming out of our core team with MIT, so the funding this time is focused on making the solution more robust, as well as bringing on the team that works with the clients and partners.
We can solve a lot of problems but are staying focused on solving just a few meaningful ones through time-series super apps. We know that every company runs on four time series, so the goal is covering these in depth and with speed: things like sales forecasting, consumption forecasting, discount forecasting, how to sunset products, catalogue optimisation, etc. We’re excited and looking forward to putting GenAI for tabular data into the hands of as many customers as possible.
Want to learn more about AI and big data from industry leaders? Check out AI & Big Data Expo taking place in Amsterdam, California, and London. The comprehensive event is co-located with other leading events including BlockX, Digital Transformation Week, and Cyber Security & Cloud Expo.
Explore other upcoming enterprise technology events and webinars powered by TechForge here.
Be the first to comment