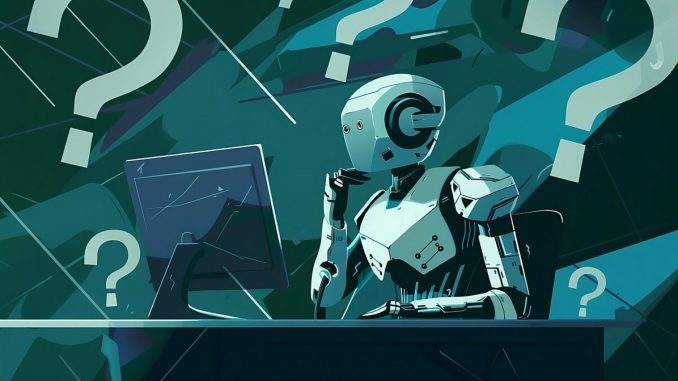
Join us in returning to NYC on June 5th to collaborate with executive leaders in exploring comprehensive methods for auditing AI models regarding bias, performance, and ethical compliance across diverse organizations. Find out how you can attend here.
Over the past 10 years, the data tooling and infrastructure world has exploded. As the founder of a cloud data infrastructure company in the early days of cloud computing in 2009, plus the founder of a meetup community for the nascent data engineering crowd in 2013, I found a place at the center of this community even before âdata engineerâ was a job title. It is from this seat that I can reflect on the lessons learned from our recent data tooling past and how it should guide development of a new AI era.
In tech anthropology, 2013 was a period between the âbig dataâ era and the âmodern data stackâ era. In the big data era, as the name suggests, more data was better. Data was purported to contain the analytical secrets to unlock new value in a business.
As a strategic consultant for a large internet company, I was once tasked to build a plan to chew through the data exhaust from billions of DNS queries per day and find a magical insight buried in this that could become a new line of business for the company worth $100 million. Did we find this insight? Not in the relatively short time (months) we had to spend on the project. As it turns out, storing big data is relatively easy, but generating big insights takes significant work.Â
But not everyone realized this. All they knew was that you couldnât play the insights game if your data house wasnât in order. So, companies of all shapes and sizes rushed to beef up their data stacks, causing an explosion in the number of data tools offered by vendors who proposed that their solution was the missing piece of a truly holistic data stack that could produce the type of magic insight a business was looking for.
VB Event
The AI Impact Tour: The AI Audit
Request an invite
Note that I donât use the term âexplosionâ lightly â in the recent MAD (Machine Learning, AI and Data) Landscape of 2024, author Matt Turck notes that the number of companies selling data infrastructure tools and products in 2012 (the year he started building his market map) was a lean 139 companies. In this yearâs edition, there are 2,011 â a 14.5X increase!
A couple things happened that helped shape the current data landscape. Enterprises began to move more of their on-premise workloads to the cloud. Modern data stack vendors offered managed services as composable cloud offerings that could offer customers more reliability, better flexibility of their systems and the convenience of on-demand scaling.
But as companies barreled through the zero interest rate policy (ZIRP) period and expanded their number of data tooling vendors, cracks started to emerge in the MDS facade. Issues of system complexity (brought on by many disparate tools), integration challenges (numerous different point solutions that need to talk to each other) and underutilized cloud services left some wondering whether the promise of the MDS panacea would be achieved.
Many Fortune 500 companies had invested heavily in data infrastructure without a clear strategy for how to generate value from that data (remember, finding insights is hard!), leading to inflated costs without proportional value. But it was trendy to collect various tools â one would often hear reports of multiple overlapping tools being used by different teams at the same company. Across business intelligence (BI) for instance, many companies would have Tableau, Looker and perhaps even a third tool installed that essentially served the same business purpose while racking up bills three times as fast.
Of course this type of excess would ultimately end with the ZIRP bubble popping. Yet, the MAD landscape has not shrunk but continues to grow. Why?
What is the new âAI stack?â
Obviously, many of the data tooling companies were so well capitalized during ZIRP that they will be able to continue operating in the face of tough enterprise budgets and market demand for their services decreasing. One reason is that there still isnât much churn, produced by startup failure or consolidation, to be seen in the number of logos.
But the main reason is the rise of the next wave of data tooling fueled by the boom of interest in AI. What is somewhat unique is that this new AI wave picked up steam before any real market shake out or consolidation from the last wave (MDS) was complete, producing even more new data tooling companies.
Yet, if one believes, as I do, that the âAI stackâ is a fundamentally new paradigm, then this is somewhat understandable. At a high level, AI is driven by massive amounts of unstructured data (think of internet-sized piles of text, images and video) while the MDS was built for smaller amounts of structured data (think tabular data in spreadsheets or databases).
Further, the so-called non-deterministic or âgenerativeâ nature of AI models is completely different from the deterministic approach designed into more traditional machine learning (ML) models. These older models were often designed to predict outcomes based on a limited set of training data. But the new generative AI models are designed to synthesize summaries or generate insights â meaning that their output can be different each time the model is run even though the inputs havenât changed. To prove this, note the difference youâll get from ChatGPT when asking it an identical question two or more times.
Since the architecture and output of AI models is fundamentally different, developers must adopt new paradigms to test and evaluate such responses according to the original intent of the user or application. Not to mention guaranteeing the ethical safety, governance and monitoring of AI systems. Some of the additional areas around the new AI stack that warrant further investigation are agent orchestration (AI models talking to other models); opportunities around smaller, purpose-built models for vertical use-cases bringing disruption to traditional industries that have been too expensive and complex to automate; and workflow tools that enable the collection and curation of fine-tuning datasets which enterprises can use to âinsertâ their own private data to create customized models.
All these opportunities and more will be addressed as part of the new AI stack as new developer platforms emerge. Hundreds of startups are already working on these challenges by building â you guessed it â a fresh batch of state of the art tools.
How can we build better and smarter this time around?
As we enter this new âAI era,â I think itâs important that we acknowledge where we came from â after all, data is the mother of AI and the myriad of data tools in recent history at a minimum provided a solid education to get businesses on a firm path of treating their data as a first class citizen. But Iâm left asking myself: âHow can we avoid the tooling excesses of the past as we continue to build towards our AI future?â
One suggestion is for enterprises to fight to develop clarity around the specific value they expect a particular data or AI tool to give to their business. Overinvestment in technology trends for the wrong reasons is never a good business strategy, and while AI is currently sucking all the air out of the room â and the money out of corporate IT and software budgets â itâs important to focus on deploying tools that can demonstrate clear value and actual ROI.Â
Another appeal would be to founders to stop building âme tooâ data and AI tool options. If there are already multiple tools in the market that youâre considering entering, take the time to ask yourself: âAre we the absolute best founding team with unique and differentiated experience that drives a key insight in the way weâre attacking this problem?â If the answer isnât a resounding yes, donât pursue building that tool â no matter how much money VCs are willing to throw at you.Â
Finally, investors would be advised to think carefully about where value will likely accrue at various layers of the data and AI tooling stack prior to investing in early stage companies. Too often, I see VCs with a single checkbox criteria â if the tool-building founder has a certain pedigree or comes out of a particular tech company, they write them a check immediately. This is lazy, plus it produces too many undifferentiated data tools crowding the market. No wonder we need a magnifying glass to read MAD 2024.
A speaker at a recent conference suggested businesses ask themselves âwhatâs the cost to your business if a single row of your data is inaccurate?â That is to say, can you establish a clear method of articulating a framework around how you quantify the value of data, or a data tool, in your business?Â
If we canât get even that far, no amount of budget spent or venture capital invested in data and AI tooling will solve our confusion.Â
Pete Soderling is founder and general partner of Zero Prime Ventures.Â
DataDecisionMakers
Welcome to the VentureBeat community!
DataDecisionMakers is where experts, including the technical people doing data work, can share data-related insights and innovation.
If you want to read about cutting-edge ideas and up-to-date information, best practices, and the future of data and data tech, join us at DataDecisionMakers.
You might even consider contributing an article of your own!
Read More From DataDecisionMakers
Be the first to comment